ByteDance AI Analysis Introduces StemGen: An Finish-to-Finish Music Era Deep Studying Mannequin Educated to Hearken to Musical Context and Reply Appropriately
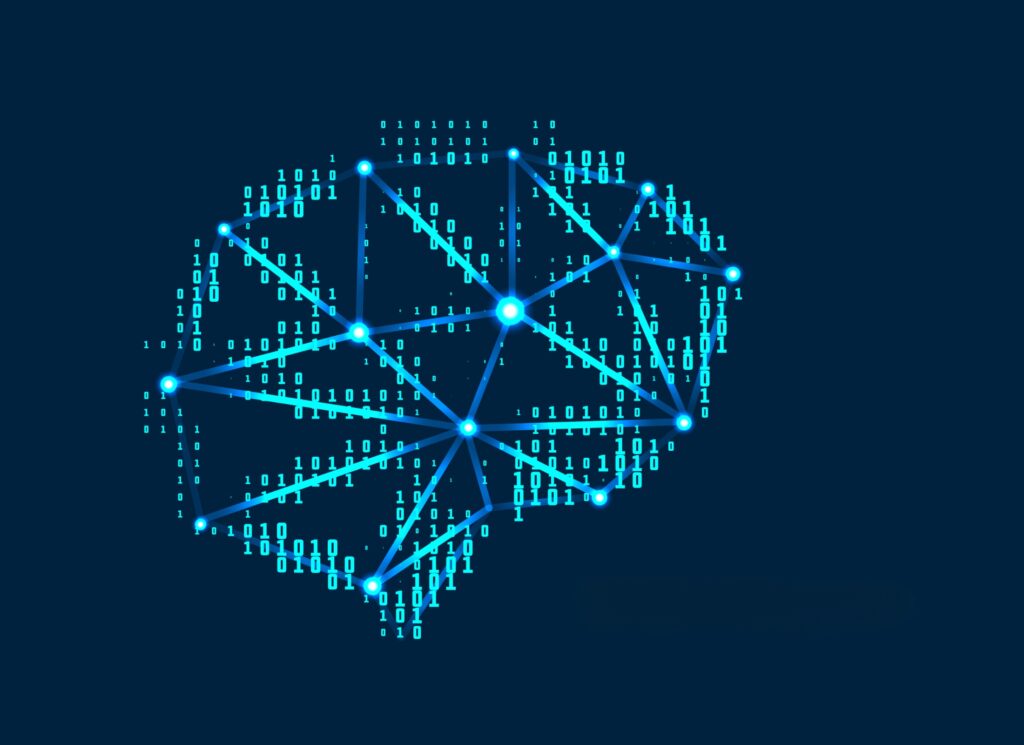
Music era utilizing deep studying entails coaching fashions to create musical compositions, imitating the patterns and constructions present in present music. Deep studying strategies are generally used, similar to RNNs, LSTM networks, and transformer fashions. This analysis explores an revolutionary method for producing musical audio utilizing non-autoregressive, transformer-based fashions that reply to musical context. This new paradigm emphasizes listening and responding, in contrast to present fashions that depend on summary conditioning. The examine incorporates latest developments within the area and discusses the enhancements made to the structure.
Researchers from SAMI, ByteDance Inc., introduce a non-autoregressive, transformer-based mannequin that listens and responds to musical context, leveraging a publicly accessible Encodec checkpoint for the MusicGen mannequin. Analysis employs customary metrics and a music info retrieval descriptor method, together with Frechet Audio Distance (FAD) and Music Data Retrieval Descriptor Distance (MIRDD). The ensuing mannequin demonstrates aggressive audio high quality and sturdy musical alignment with context, validated by means of goal metrics and subjective MOS exams.
The analysis highlights latest strides in end-to-end musical audio era by means of deep studying, borrowing strategies from picture and language processing. It emphasizes the problem of aligning stems in music composition and critiques present fashions counting on summary conditioning. It proposes a coaching paradigm utilizing a non-autoregressive, transformer-based structure for fashions that reply to musical context. It introduces two conditioning sources and frames the issue as a conditional era. Goal metrics, music info retrieval descriptors, and listening exams are needed for mannequin analysis.
The tactic makes use of a non-autoregressive, transformer-based mannequin for music era, incorporating a residual vector quantizer in a separate audio encoding mannequin. It combines a number of audio channels right into a single sequence ingredient by means of concatenated embeddings. Coaching employs a masking process, and classifier-free steering is used throughout token sampling for enhanced audio context alignment. Goal metrics assess mannequin efficiency, together with Fr’echet Audio Distance and Music Data Retrieval Descriptor Distance. Analysis entails producing and evaluating instance outputs with actual stems utilizing varied metrics.
The examine evaluates generated fashions utilizing customary metrics and a music info retrieval descriptor method, together with FAD and MIRDD. Comparability with actual stems signifies that the fashions obtain audio high quality corresponding to state-of-the-art text-conditioned fashions and show sturdy musical coherence with context. A Imply Opinion Rating check involving contributors with music coaching additional validates the mannequin’s capability to supply believable musical outcomes. MIRDD, assessing the distributional alignment of generated and actual stems, offers a measure of musical coherence and alignment.
In conclusion, the analysis performed will be summarized in beneath factors:
- The analysis proposes a brand new coaching method for generative fashions that may reply to musical context.
- The method introduces a non-autoregressive language mannequin with a transformer spine and two untested enhancements: multi-source classifier-free steering and causal bias throughout iterative decoding.
- The fashions obtain state-of-the-art audio high quality by coaching on open-source and proprietary datasets.
- Customary metrics and a music info retrieval descriptor method have validated the state-of-the-art audio high quality.
- A Imply Opinion Rating check confirms the mannequin’s functionality to generate sensible musical outcomes.
Take a look at the Paper and Project. All credit score for this analysis goes to the researchers of this challenge. Additionally, don’t overlook to hitch our 34k+ ML SubReddit, 41k+ Facebook Community, Discord Channel, and Email Newsletter, the place we share the most recent AI analysis information, cool AI tasks, and extra.
If you like our work, you will love our newsletter..
Good day, My title is Adnan Hassan. I’m a consulting intern at Marktechpost and shortly to be a administration trainee at American Specific. I’m at present pursuing a twin diploma on the Indian Institute of Know-how, Kharagpur. I’m keen about know-how and wish to create new merchandise that make a distinction.